There’s no ‘one-size-fits-all’ solution to a GenAI hosting strategy. Whether you are running these disruptive applications on the cloud or on-premise, there are pros and cons to each. But the fundamental driver of this decision will be the use case, and your hosting strategy must start there.
Where you host your GenAI service – on the edge or the cloud – is a decision that is generally made for you, rather than by you. It’s an equation – you punch in the variables, and the answer is provided. The dimensions under your control are how you make the best of that situation.
The variables are:
- The volume of data with which you have to work and the compute capabilities required to process it – the larger the volumes, the more likely you are to need the power of the cloud.
- The capabilities of your infrastructure – whatever your intentions, you will need a high-speed, high-capacity network to connect data to the cloud. If that doesn’t exist – or if you cannot access it – then edge computing is your only option.
- The need for real-time data – no matter how good your network, gathering data from where it is sourced, transferring it to the cloud and sending it back again takes time (latency). If your use case depends on instantaneous insights, then it must be processed at the edge.
- Resiliance and security – Where this is the case, edge hosting would ensure that the service is available even if there is no or limited external connectivity and that sensitive data isn’t transferred externally.
In the technology environments to which GenAI will be applied, there are two major domains – the world of Information Technology (IT) and that of Operational Technology (OT). Generally, the IT world is more likely to be suitable for cloud-based GenAI deployments, while the OT world will be better adapted to edge-based solutions. Ultimately, however, it is the use cases that will make the difference. Motor vehicle applications provide a good example of how different use cases influence hosting strategies.
Google Waze is a community-driven navigation app that uses real-time data submitted by its users to provide the fastest route to a destination, taking into account traffic jams, accidents, road hazards, and other obstacles reported by fellow drivers. If the results take a few seconds to reach the driver, then there is no loss of value for the service. As a result, Waze data is processed on the cloud – a big brain (Large Language Model – LLM) at the centre that serves millions of users. By contrast, an autonomously driven vehicle, which may use dozens of cameras to inform driving decisions, must have results in milliseconds – so the data processing must take place locally – a small brain (Small Language Model – SLM) at the edge serving an individual vehicle.
In another example of a use case demanding edge processing, Orange Business is working on a safety-related AI use case for a mining company. This requires split-second decision-making, and the underground location makes it hard to install an IT infrastructure. Consequently, hosting this service at the edge was the obvious solution. GenAI solutions for Smart Factory environments that may have connectivity challenges are another example of a situation where edge processing is preferable – particularly if these use cases are safety-related.
By contrast, if you have a Co-Pilot use case in a well-connected office environment where there is no need for instantaneous results, then it makes perfect sense to host the service on the cloud.
The big brain at the centre
Cloud computing models offer many advantages for GenAI services.
First of all, they generally operate on a pay-as-you-go model, reducing upfront costs and allowing for flexible resource allocation. Where appropriate, you will find that the aggressive pricing of the Hyperscalers makes hosting your GenAI service with them a compelling proposition. (Although there are ways of further optimising your costs if you go down this route – see our White Paper, ‘A Hungry Mouth to Feed: Addressing the skyrocketing costs of AI services’ for more details on this.)
It also offers vast computing resources and storage capacity, allowing for easy scaling of AI models and handling large datasets, together with access to cutting-edge hardware like GPUs and specialised AI accelerators. And, of course, the management of the service is entirely offloaded to the cloud provider.
However, as we’ve discussed, there are latency issues which may be unacceptable for real-time applications. There are also very legitimate privacy and security concerns, particularly relating to data sovereignty: and transferring large amounts of data to and from the cloud can require significant network capacity (and may incur data ingress/egress charges.)
Lots of little brains at the edge
Equally, edge computing has much to recommend it. Many of these benefits are corollary to the points made above.
Sensitive data is stored and processed locally, reducing the risk of breaches during transmission and storage in the cloud and simplifying compliance concerns. This is particularly important for healthcare, finance, and personal data applications.
Processing data closer to the source reduces latency, results in faster response times and also lowers bandwidth costs. More significantly, edge AI requires only limited connectivity, making it suitable for remote locations, areas with unreliable networks, and situations where constant connectivity is not guaranteed.
On the minus side, edge devices typically have less processing power than cloud servers, restricting the complexity of AI models that can be deployed. There are also CapEx costs attached to building and scaling your digital infrastructure, rather than simply accepting the OpEx costs of consuming existing cloud services. And, of course, the responsibility of managing this infrastructure falls to you.
Big Brian or Little Brain – or both?
While the choice of cloud or edge hosting may be forced upon you to a large extent, you still have a choice in how you structure that service.
Take, for example, a situation such as field service in which you have employees operating in network environments that are not under your control. These field operatives may only have access to a mobile phone that cannot deliver either the connectivity required to hook up with a big brain or the local processing power to serve as a small brain. In this case, you might use the phone to capture the serial number of the machine that needs to be serviced – this can then be sent via text to a central facility and the requisite information (schemas, manual pages, etc) sent back to the mobile phone.
As a side note, the importance of natural language interaction with GenAI models has been hugely underestimated. For many use cases, particularly those involving front-line workers, employees neither have access to keyboards nor are particularly used to using them as part of their day-to-day working lives. For example, we are working with a police department on providing a voice interface to an AI system that will record the basic information about an incident at the scene: this will then be used as the basis for the automatic generation of a report – saving hours in the working week of every officer. This is a great example of a small brain and a large brain working together to generate the efficiencies that every organisation is striving for.
Conclusion
The use case may dictate where you host your GenAI service, but you still have a lot of discretion in terms of how you operationalise that capability. Price and performance considerations both dictate that you should use the smallest model available to you in each given use case. A hybrid approach combining edge and cloud computing can often be optimal – edge devices can handle real-time processing and data filtering, with the cloud used for complex model training, data storage, and analytics.
These are, undoubtedly, important decisions, but those responsible for GenAI strategy in their companies often agonise unnecessarily about which route to take. Fundamentally, if you focus on the use case, it will lead you to the right decision.
GenAI is going to change your world, and if you are worried about the risks of making a decision, those risks are minuscule in comparison to making no decision at all. As the Roman statesman Cicero observed more than two millennia ago, “More is lost by indecision than wrong decision". So make yours sooner rather than later.
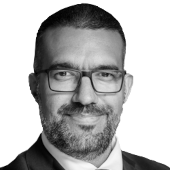
Michaël Deheneffe has been supporting major European companies in their digital transformation for 25 years. He has led programs that have helped major groups transform and take market leadership. It combines 3 inseparable factors: corporate strategy, data and AI, and ethics. Michaël is VP of Data and AI strategy at Orange Business.